In the artificial intelligence world, there exist two famous kinds of AIs: generative and predictive. Both have unique capabilities and applications that formulate them into very vital tools in various industries. This post looks into the main differences between Generative AI and Predictive AI in an attempt to explain their different functionalities and benefits.
What is Generative AI?
Generative AI would then refer to systems that could create new content. These AI models are trained with vast datasets and run by machine learning algorithms to generate text, images, audio, and other forms of media. Examples include GPT-3 for generating text and DALL-E for generating images.
Generative AI Applications
Generative AI has wide applications in creative industries. It is capable of creating unique artworks, writing articles, and even composing music. The system can also generate quite realistic human faces. In marketing, generative AI creates personalized content for the advertisement targeting campaign.
What is Predictive AI?
Predictive AI involves the prediction of events in the future based on past data. It makes predictions accurate with the application of statistical models and machine learning algorithms pinpointing trends and patterns. Common fields of application are finance, healthcare, and retail.
Applications of Predictive AI
In finance, predictive AI models project stock prices and market trends. In healthcare, they predict which diseases will break out and the results for patients. Retailers use predictive AI to anticipate customer behavior and optimize inventory management.
Key Differences Between Generative AI and Predictive AI
Purpose and Functionality
Generative AI creates new content, while predictive AI predicts the occurrence of future events. On this basis, generative AI applies better in areas of creation, while predictive AI does best when it analyzes data to drive a decision.
Data Requirements
Generative AI requires large datasets from which it learns to generate new content. Predictive AI, however, uses smaller, highly-focused datasets to deliver highly accurate predictions.
Output
Generative AI generates outputs that are novel and creative in nature—for instance, text, images, music. Predictive AI, however, provides the business with forecasts and insights from the past data so that it may act wisely.
Use Cases
Generative AI finds applications in content creation, art, and entertainment; Predictive AI finds its applications in finance, health, and retail by fast-forwarding the future and making decisions accordingly.
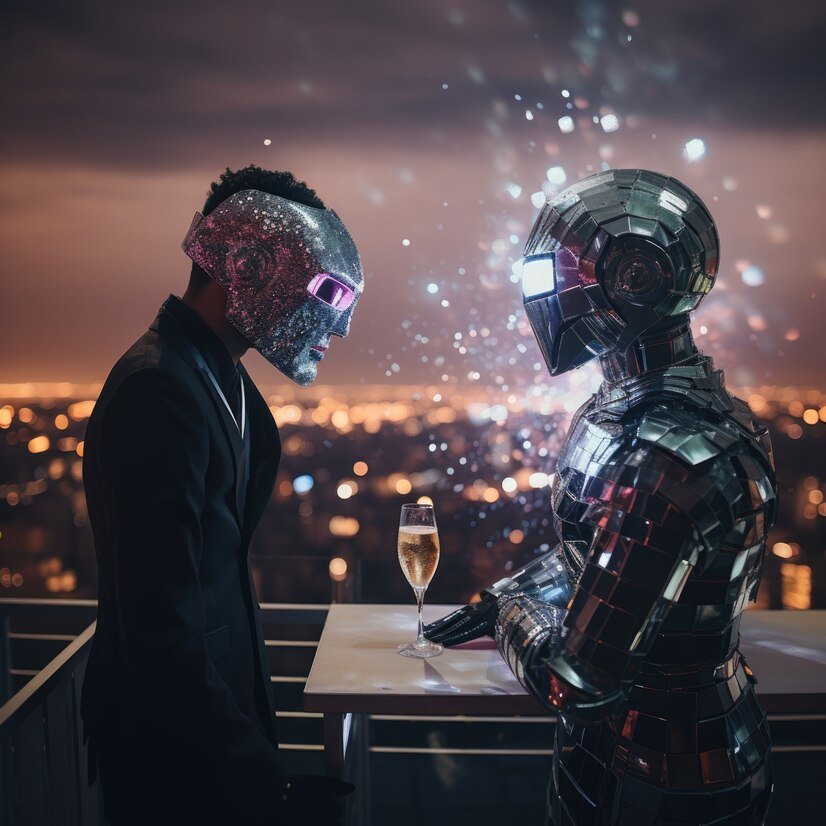
Challenges and Limitations
Generative AI Challenges
Generative AI models sometimes replicate biased or inappropriate content when they pick it up from the bulk of data they get trained on. Making sure that it is ethically used and reducing the biases is quite a challenge.
Predictive AI Challenges
Predictive AI depends on the quality and accuracy of past data. Any inaccurate or incomplete data may lead to incorrect predictions, hence affecting decision-making processes.
Future Prospects
Generative AI Advancements
Generative AI is getting evolved with improvements in deep learning and neural networks. Further innovations could lead to more and more impressive content creation.
Predictive AI Advancements
Predictive AI is also undergoing rapid development with improvements in data analysis and machine learning algorithms. These will enable predictions to be made with a greater degree of accuracy and reliability and will benefit a wide range of industries.
Conclusion
To understand how best to leverage both generative and predictive AI, one must know what sets them apart. Generative AI comes into play during the creation of new content, while predictive AI serves to guide in decision-making. Both kinds of AI are truly powerful transformers of innovation and efficiency across a number of sectors. As technology advances further, the future will lie in integration with generative and predictive AI.